Enjoy DatePsychology? Consider subscribing at Patreon to support the project.
As men grow increasingly concerned with their physical appearance, an effect driven by social media and dating apps, a novel service has emerged — facial attractiveness ratings. For a low fee you can get someone to rate your face. These are all over the place: “blackpill” YouTubers are doing it, Reddit has a “rate me” forum, and you can find these services on freelancer websites.
Many people have asked me: “Do they work? How accurate are they?”
My answer is “maybe, kinda” — having a single person rate your face is going to give you very limited information.
How Much Do We Agree On Attractive Faces?
Past research has found high agreement (.9) on facial attractiveness ratings (Langolis et al., 2000) using Cronbach’s alpha. When I began researching facial attractiveness this was my initial impression: we agree a lot on what an attractive face is. This was a belief I formed based on the high Cronbach’s alpha coefficient. However, it turns out Cronbach’s alpha may not be well suited for demonstrating agreement on facial ratings (Hönekopp, 2006).
Instead, intraclass correlation coefficients (ICCs) for individual subjects may reflect what is going on better. These are consistently much lower. Further, they tend to be lower for women than for men. Hönekopp (2006) found an ICC for women at .39 and Roth et al. (2023) found female agreement at only .25 and .5 for men. When I ran a series of faces from the CFD I found an ICC at .27 for women and .32 for men, despite a Cronbach’s alpha of .9 and .89 for the two sexes of raters respectively.
You can see what is going on when you look at the actual distribution of ratings from a group of raters. A face with an average score of 5/10 might receive a substantial number of participants who rate them as a 2 and a substantial number who rate them as an 8.
Here we can see this in Hönekopp (2006):
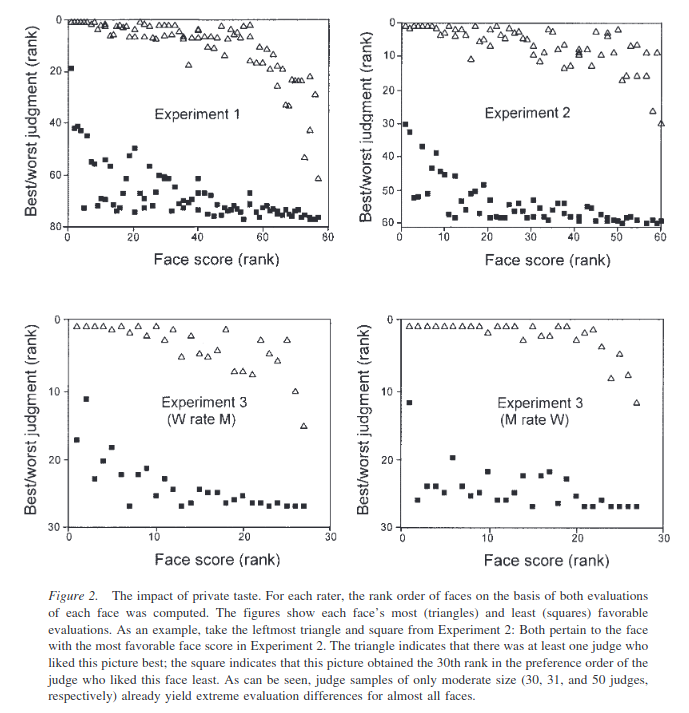
The images above show the highest and lowest ratings most faces received. The triangles are the highest rank of face received and the squares are the lowest rank. In essence, most faces will be ranked highly by some people and low by some people.
Here you can see what happens with an individual face:
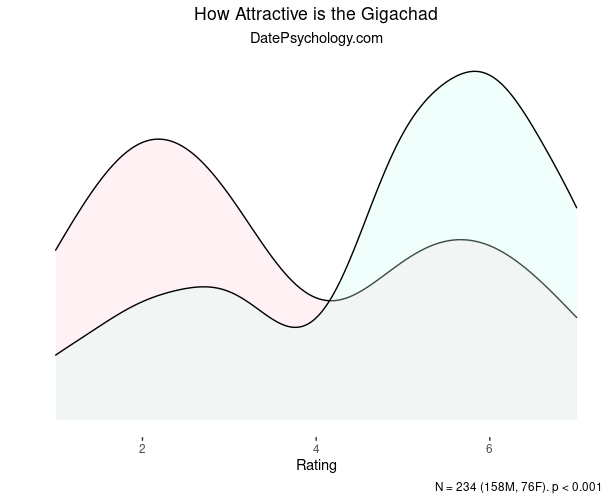
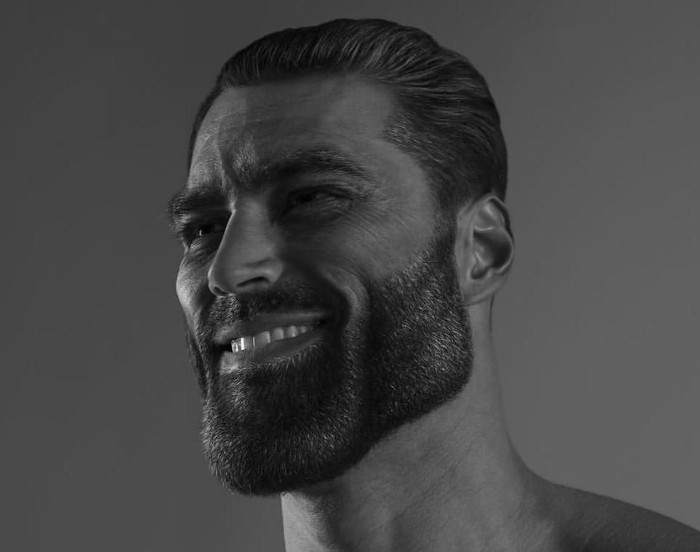
The average rating by women for the Gigachad was a 3.58 and for men was a 4.88 (on a 7 point Likert scale). However, the distributions show substantial disagreement within both male and female raters. Some participants found him to be very high in attractiveness while some participants found him to be very low in attractiveness.
Below are four histograms for the ratings of single faces from the CFD:
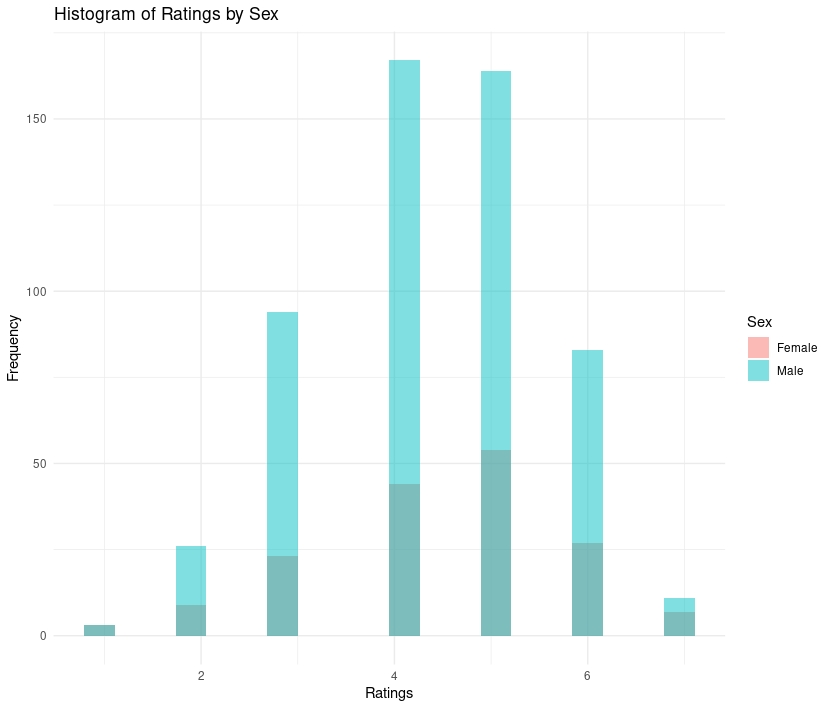
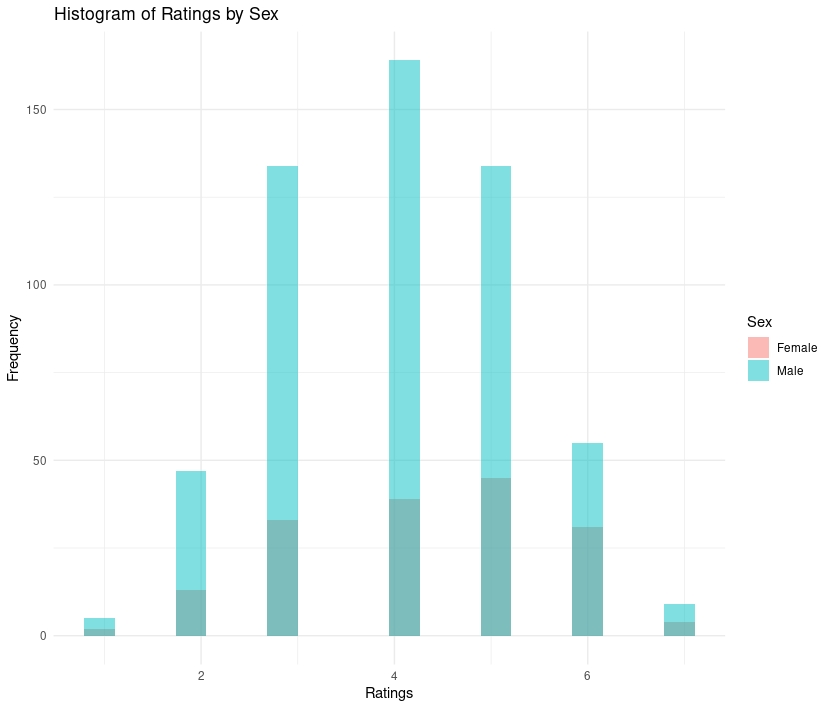
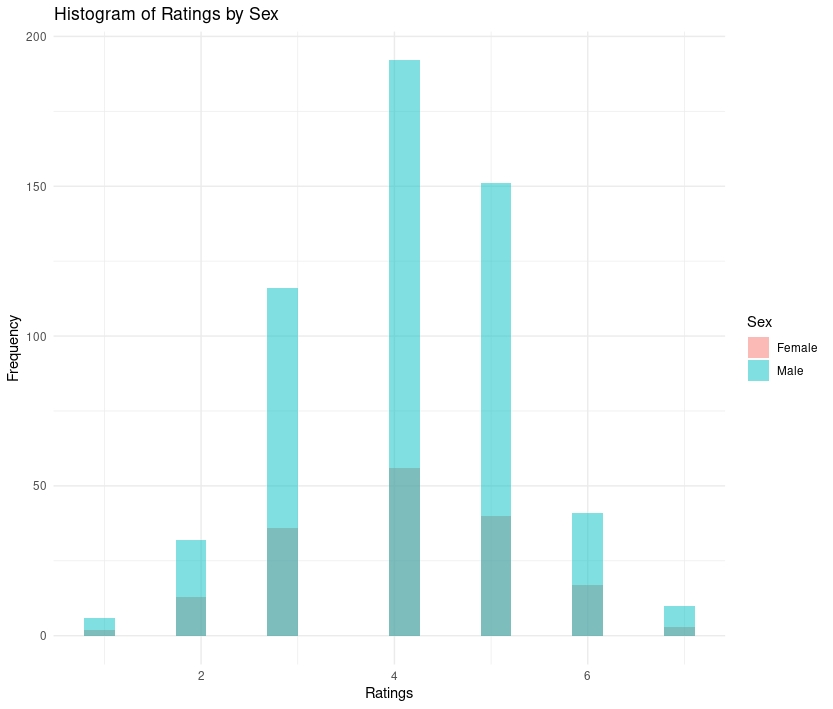
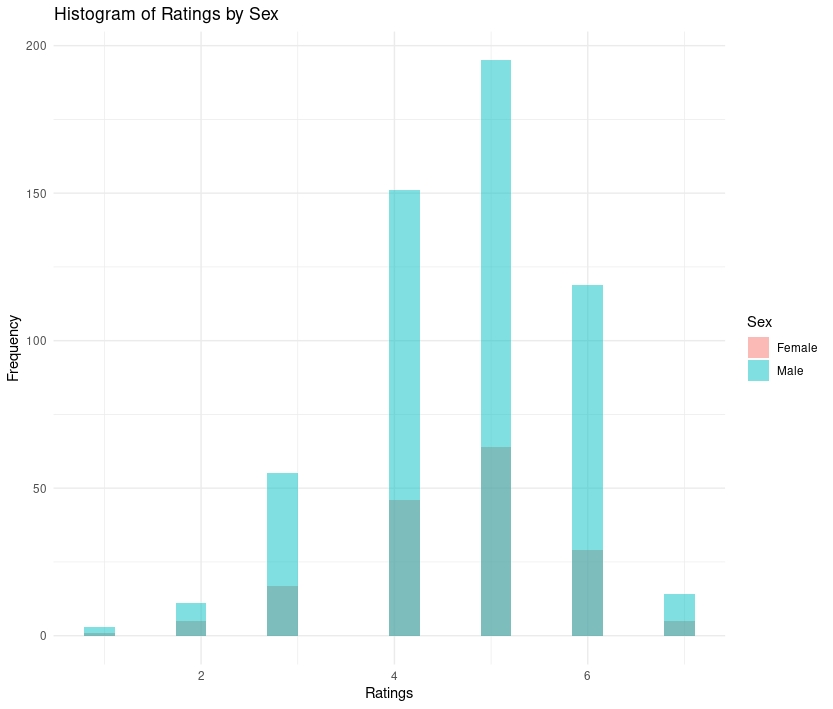
The average ratings of these faces were 4.7, 4.1, 4.1, and 4.4 on a scale of 7. However, a substantial number of participants still rated these faces above and below the average.
This brings us to an important point: you are not necessarily your average rating.
Limitations of Attractiveness Rating Services
First, we have seen that individual raters can fall anywhere. You might think that someone is an expert on faces (they probably claim to be). They may even be very good at identifying what your average rating might be (although you can’t know if they are). We have algorithms that can do this: they can produce a single number that is close to the average rating of a human sample (because they are trained on human raters). Maybe some people can, too, but the information provided by a single number is very limited.
Look again at the distributions in the previous section: the average score was a 4. Yet, most individual raters did not consider those faces to be a 4. Are you really a 4 if most people don’t think you are? Maybe your average score isn’t the best way to think about how attractive you appear to other people.
Very few facial rating services use samples to give you a score at all, so they are limited by the high individual variation we see in attractiveness ratings. An exception is Photofeeler, where you can upload pictures and receive ratings by samples. However, Photofeeler is also limited in that it only tells you an average number. You don’t get to see your distribution of ratings.
For services that do rely on a single “expert” to rate your face, take it with a big grain of salt, even if you think they know faces. One thing we know about human psychology is that we aren’t able to check our own biases very well (even when we are aware of them). There is no way of knowing at all how well their rating will reflect the ratings of large groups (how most people see you). Even if they can give you a single number that resembles a sample average you’d still be missing a lot of information about how most people see you. To know this you need to look at a distribution from a group of raters.
References
Hönekopp, J. (2006). Once more: is beauty in the eye of the beholder? Relative contributions of private and shared taste to judgments of facial attractiveness. Journal of Experimental Psychology: Human Perception and Performance, 32(2), 199.
Langlois, J. H., Kalakanis, L., Rubenstein, A. J., Larson, A., Hallam, M., & Smoot, M. (2000). Maxims or myths of beauty? A meta-analytic and theoretical review. Psychological bulletin, 126(3), 39
Roth, T. S., Samara, I., Perea-Garcia, J. O., & Kret, M. E. (2023). Individual attractiveness preferences differentially modulate immediate and voluntary attention. Scientific Reports, 13(1), 2147.
3 comments
“However, Photofeeler is also limited in that it only tells you an average number. You don’t get to see your distribution of ratings.”
This isn’t true though? I have used Photofeeler recently, and they absolutely give you the distribution of scores. They even have helpful information like how “tough” the person who gave you a particular score is (by “tough” I just mean does the grader tend to give lower or higher scores on average).
Also: the importance of variance (and not just mean) is received wisdom in the more data-centric parts of the manosphere. One of the most commonly given pieces of advice is to be polarizing: you need to specialize your brand, focusing on a particular demographic who will find you *really* attractive. “Be polarizing” has been in the water supply for a while, but I know for sure that Christian Rutter talked about it in his famous book *Dataclysm* where he mentioned that profiles with a higher variance of scores tended to get more replies to messages.
Hi Alex. Are there any study on the correlation between attractiveness and political leanings?
My personal is that it follows a reverted-J curve : people on the right are more attractive than people on the left, but people on the FAR-right are strongly less attractive than anyone else.
Photofeeler does give the distribution.